Effective Visualization of Multi-Dimension Image Data in Python
Multi-dimensional image data is, generally speaking, cumbersome to visualize.
In scientific imaging (or in most imaging areas), multi-dimensional images are very common. The additional dimension could be anything from the physical 3rd dimension ("Z axis"), where 2D images are taken at different depths; to the time dimension, where 2D images are taken at different time intervals; to different channels in scientific imaging instruments such as atomic force microscopes or in RGB images.
We will use seaborn-image
, an open source image visualization library in Python based on matplotlib
.
It is heavily inspired by the popular
seaborn
library for statistical visualization
Installation
pip install -U seaborn-image
You can find out more about the
seaborn-image
project on GitHub.
Load sample 3D data
import seaborn_image as isns
cells = isns.load_image("cells")
cells.shape
(256, 256, 60)
Visualize
We will use ImageGrid
from seaborn_image
to visualize the data. It will plot a series of images on a grid.
To begin, we will only plot a few selected slices using the slices
keyword argument.
g = isns.ImageGrid(cells, slices=[10, 20, 30, 40, 50])
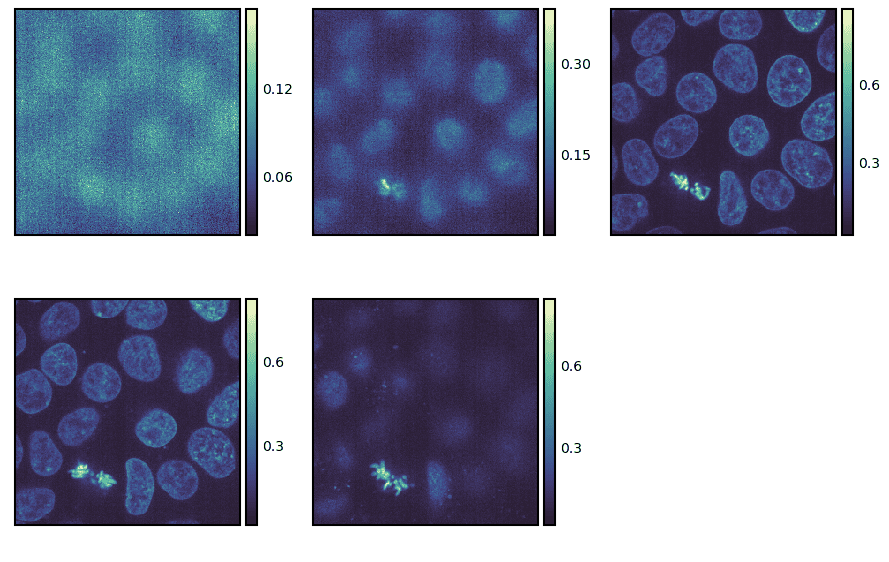
By default, the slices are taken along the last axis. However, we can take them along another dimension using the axis
keyword argument.
g = isns.ImageGrid(cells, slices=[10, 20, 30, 40, 50], axis=0)
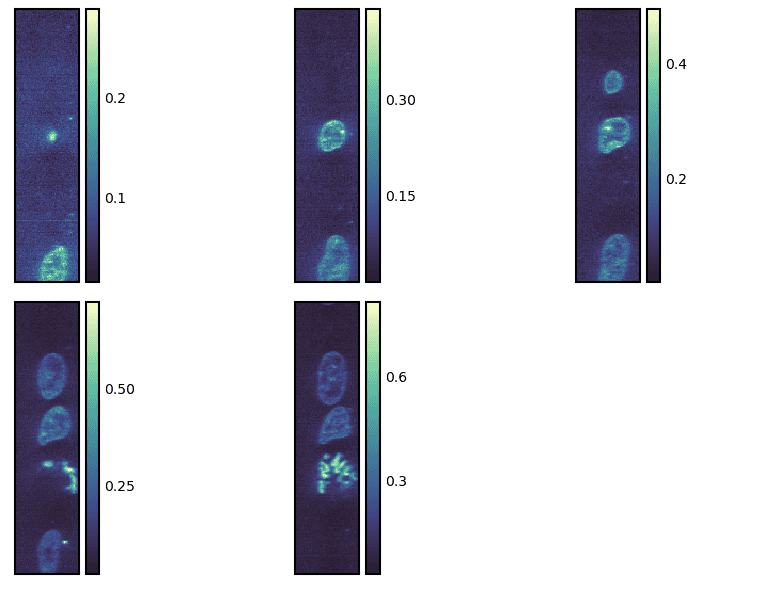
We can also specify different start/stop points as well as step sizes to take using the start
, stop
and step
parameters, respectively.
In the code below, we are starting with the 10th slice and going up to the 40th slice with steps of 3.
The slices and steps are taken over the last axis if not specified.
g = isns.ImageGrid(cells, start=10, stop=40, step=3)
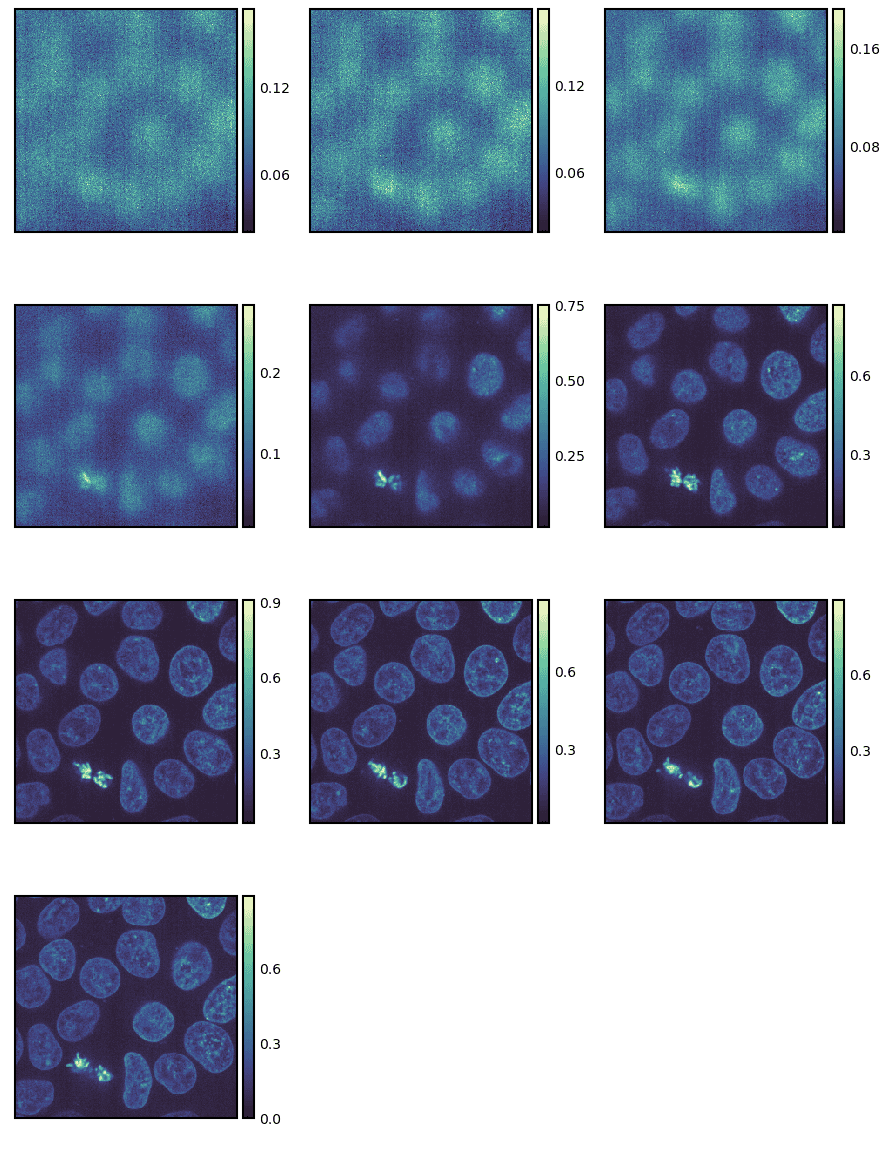
We can also just plot all the images without any indexing or slicing.
g = isns.ImageGrid(cells, cbar=False, height=1, col_wrap=10)
Note - We altered the height of the individual images and the number of image columns.
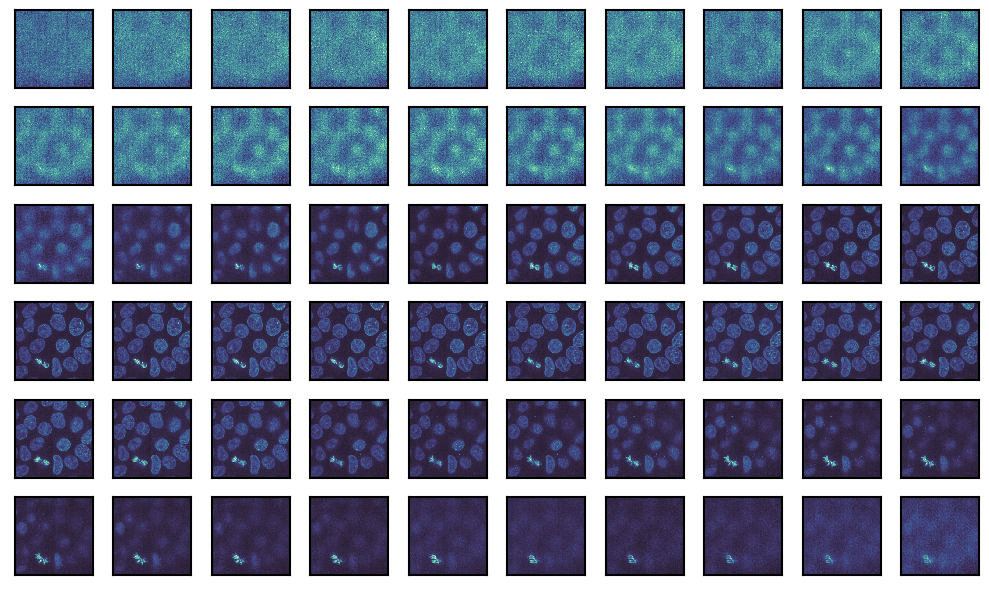
Transformations
Finally, we can also apply transformations to the image and visualize it. Here, we will adjust the exposure using the adjust_gamma
function from scikit-image
.
We can achieve this by passing the function object to the map_func
parameter. Additional parameters to the function object can be passed as keyword arguments.
from skimage import exposure
g = isns.ImageGrid(
cells,
map_func=exposure.adjust_gamma, # function to map
gamma=0.5, # additional keyword for `adjust_gamma`
cbar=False,
height=1,
col_wrap=10)
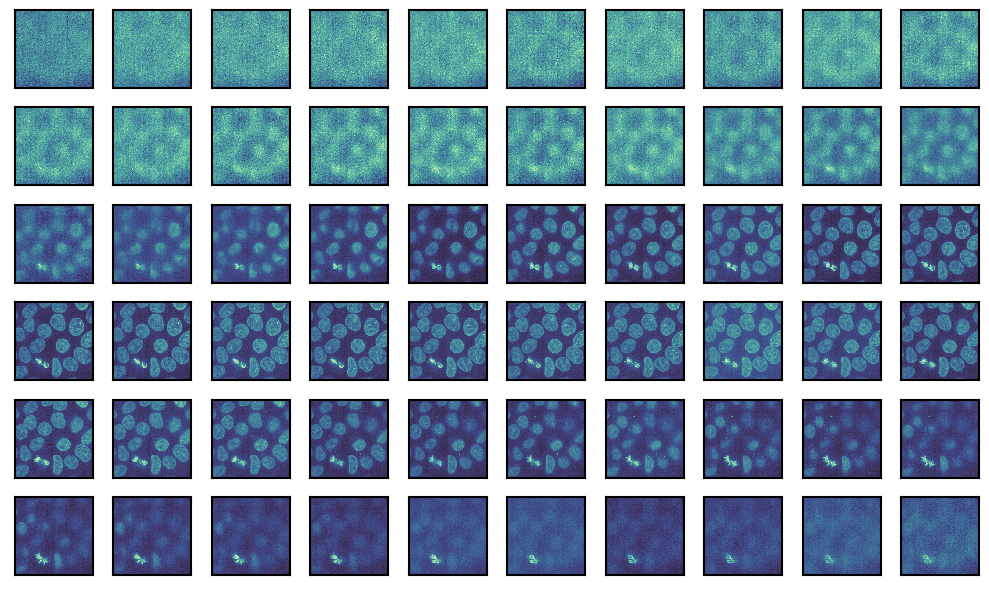
ImageGrid
returns a seaborn_image.ImageGrid
object and is a figure-level function, i.e. it generates a new matplotlib
figure. We can access the figure and all the individual axes using the fig
and axes
attributes, respectively. This means that for any customizations that are not directly available in seaborn-image
(see documentation), we can drop down to matplotlib
and use its powerful API.
Overall, as we have seen throughout this post, seaborn-image
allows us to be more productive by providing a high-level API for quick, effective and attractive image data visualization.
You can find out more about the seaborn-image
project on GitHub.
Thanks for reading!